Table of Contents
Deep learning, a subset of artificial intelligence (AI), has revolutionized how machines learn and make decisions. It involves the use of artificial neural networks with multiple layers—hence the term &8220;deep&8221; learning. This technology has become a cornerstone in the advancement of AI, driving innovations across various fields, from natural language processing to computer vision. Understanding the role of deep learning in AI reveals its profound impact on technology and society, highlighting both its potential and its challenges.
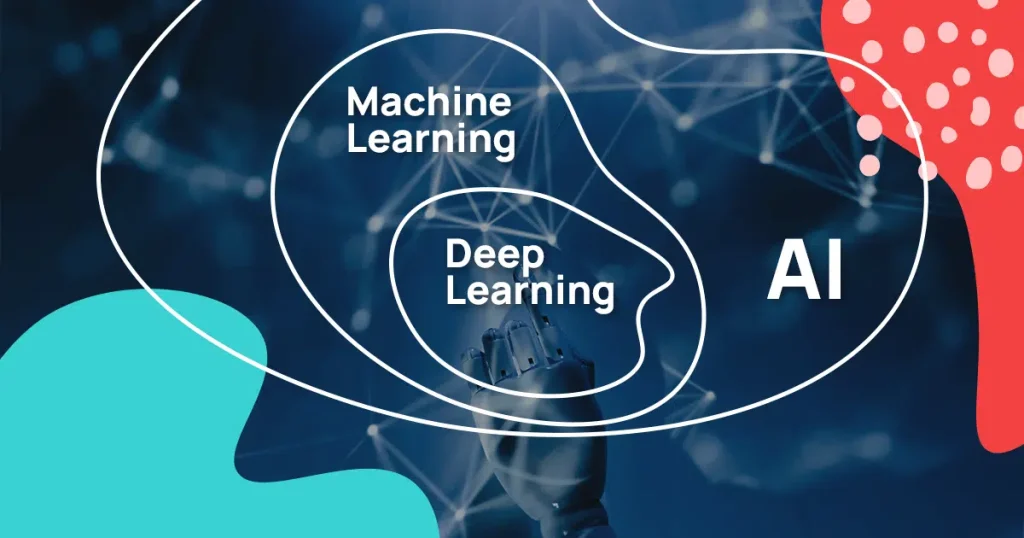
1. Understanding Deep Learning
Deep learning is a branch of machine learning that utilizes neural networks with several layers (hence &8220;deep&8221; networks) to analyze and interpret complex patterns in data. These networks consist of interconnected nodes, or &8220;neurons,&8221; that process input data through weighted connections. Each layer of the network extracts progressively higher-level features from the input, allowing the system to learn hierarchical representations.
- Artificial Neural Networks: At the core of deep learning are artificial neural networks, inspired by the human brain&8217;s structure. These networks are composed of input layers, hidden layers, and output layers. Each neuron in a layer is connected to neurons in the next layer, with each connection having an associated weight that adjusts during training to minimize prediction errors.
- Training Deep Networks: Training a deep learning model involves feeding it large amounts of labeled data and adjusting the weights of connections through a process called backpropagation. This iterative process aims to minimize the difference between the predicted output and the actual output, effectively teaching the model to make accurate predictions or classifications.
2. Applications of Deep Learning
Deep learning&8217;s ability to handle vast amounts of data and identify intricate patterns has led to breakthroughs in numerous domains:
- Natural Language Processing (NLP): Deep learning has transformed NLP, enabling machines to understand, interpret, and generate human language with unprecedented accuracy. Technologies such as language translation, sentiment analysis, and chatbots leverage deep learning models like transformers and recurrent neural networks (RNNs) to process and generate text.
- Computer Vision: In computer vision, deep learning has significantly advanced image recognition and object detection. Convolutional neural networks (CNNs) are particularly effective in analyzing visual data, enabling applications such as facial recognition, autonomous vehicles, and medical imaging analysis.
- Healthcare: Deep learning is making strides in healthcare by improving diagnostic accuracy and personalized treatment. For instance, deep learning algorithms can analyze medical images to detect diseases like cancer at early stages, predict patient outcomes, and tailor treatment plans based on individual health data.
- Finance: In the financial sector, deep learning is used for algorithmic trading, fraud detection, and risk management. Models can analyze historical data to forecast market trends, identify fraudulent transactions, and assess credit risk with high precision.
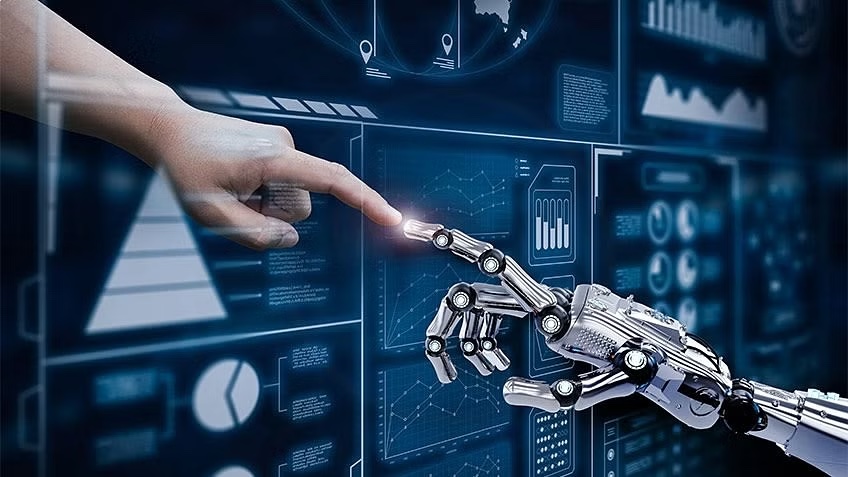
3. Challenges and Limitations
Despite its transformative impact, deep learning faces several challenges and limitations:
- Data Requirements: Deep learning models require vast amounts of labeled data to achieve high performance. Acquiring and annotating this data can be resource-intensive and may not be feasible for all applications. Additionally, the quality of the data directly affects the model&8217;s accuracy and generalizability.
- Computational Resources: Training deep learning models, especially large ones, demands significant computational power and storage. High-performance GPUs and specialized hardware are often needed to handle the intensive calculations, which can be costly and limit accessibility.
- Interpretability: Deep learning models are often described as &8220;black boxes&8221; because their decision-making processes are not easily interpretable. Understanding why a model made a specific prediction or classification can be challenging, raising concerns about transparency and accountability, particularly in critical applications like healthcare and finance.
- Bias and Fairness: Deep learning models can inadvertently learn and perpetuate biases present in the training data. This can lead to unfair or discriminatory outcomes, highlighting the need for careful consideration of data diversity and model evaluation to ensure equitable results.
4. Ethical and Societal Implications
The widespread adoption of deep learning raises important ethical and societal questions:
- Privacy: As deep learning models process vast amounts of personal data, concerns about data privacy and security arise. Ensuring that data is handled responsibly and that individuals&8217; privacy is protected is crucial in maintaining public trust.
- Job Displacement: The automation capabilities of deep learning and AI have the potential to displace certain jobs, particularly those involving repetitive tasks. While new opportunities will arise, there is a need for strategies to manage the transition and support affected workers.
- Decision-Making: The use of deep learning in decision-making processes, such as credit scoring or law enforcement, necessitates careful consideration of fairness and accountability. Ensuring that models are used ethically and that decisions are transparent is essential to prevent harm and maintain public confidence.
5. The Future of Deep Learning
The future of deep learning holds exciting possibilities and potential advancements:
- Improved Algorithms: Ongoing research is focused on developing more efficient and robust deep learning algorithms. Techniques such as transfer learning and few-shot learning aim to reduce the need for large amounts of data and improve model generalization.
- Integration with Other Technologies: Deep learning will increasingly integrate with other emerging technologies, such as quantum computing and edge computing, to enhance performance and expand applications. This integration could lead to new breakthroughs and innovations.
- Ethical AI Development: The future of deep learning will also involve addressing ethical considerations and developing frameworks for responsible AI use. Ensuring that AI systems are fair, transparent, and aligned with societal values will be a key focus for researchers, developers, and policymakers.
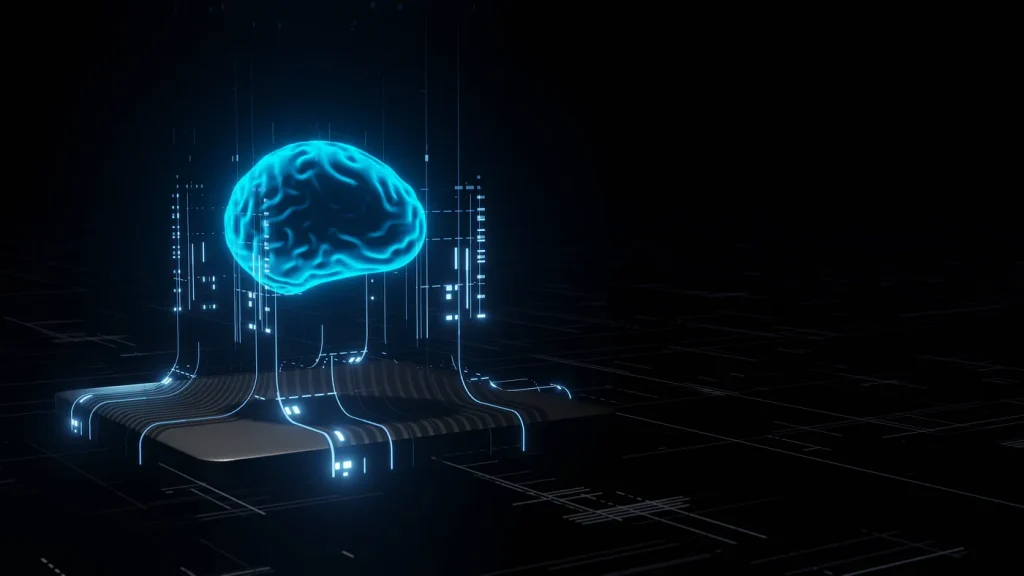
Conclusion
Deep learning has become a pivotal force in the advancement of artificial intelligence, driving innovations across a wide range of fields and applications. Its ability to analyze complex data and uncover intricate patterns has transformed technology and opened new avenues for research and development. As deep learning continues to evolve, addressing its challenges and ethical implications will be crucial in harnessing its potential for positive impact and ensuring that its benefits are widely shared. The future of deep learning promises to be both dynamic and transformative, reshaping our world in profound and unprecedented ways.